Michael Frank, a professor of cognitive and psychological sciences and director of Carney’s Center for Computational Brain Science, and Aneri Soni, a PhD candidate in Frank’s lab, have introduced a new model of human working memory, publishing on it in eLife. Their model’s simulations explain why there are limits on how much information a person can store at a time and show how the brain learns to best use the storage space it has.
Working memory allows humans to juggle different pieces of information in short-term scenarios: making a mental grocery list and then going shopping, remembering and then dialing a phone number, or studying a chess board and then making the right move. Scientists agree that the capacity of our working memory is limited to a few items at a time, but offer competing theories about how and why this is true.
According to Frank and Soni, the answer has to do with learning.
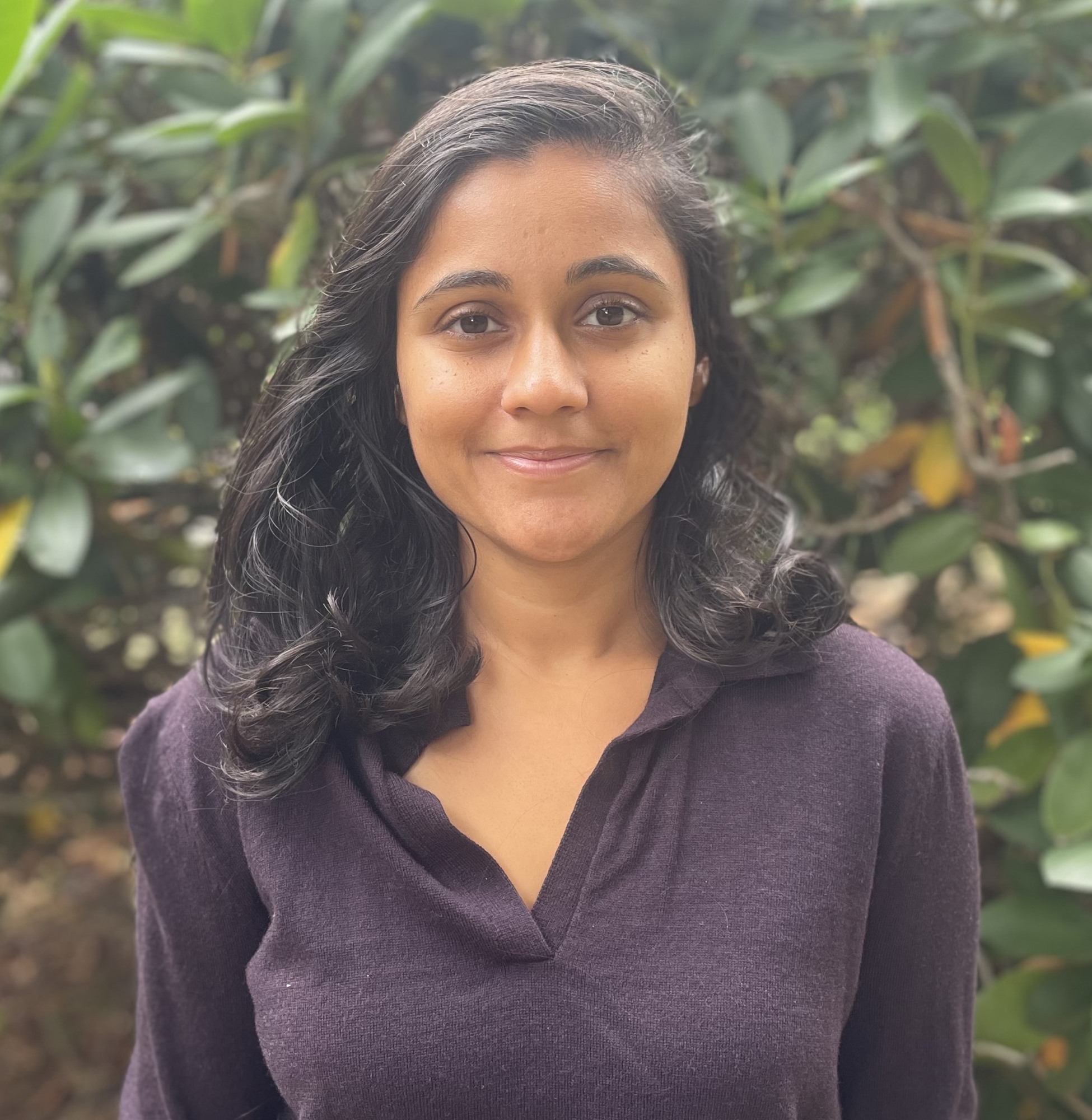
“The simulations we ran show that if we did hold more than just a few items at a time, it becomes too difficult to learn how to manage so many pieces of information at once, such that the brain gets confused and can't use the information it does store. At the same time, our new model demonstrates that, when faced with these limitations, the brain responds by learning to strategically tap into a mechanism to help conserve space,” said Soni.
Because dopamine, one of the brain’s chemical messengers, plays an important role in learning as it relates to working memory, the researchers said these findings shed new light on disorders of dopamine such as Parkinson’s disease, ADHD and schizophrenia.
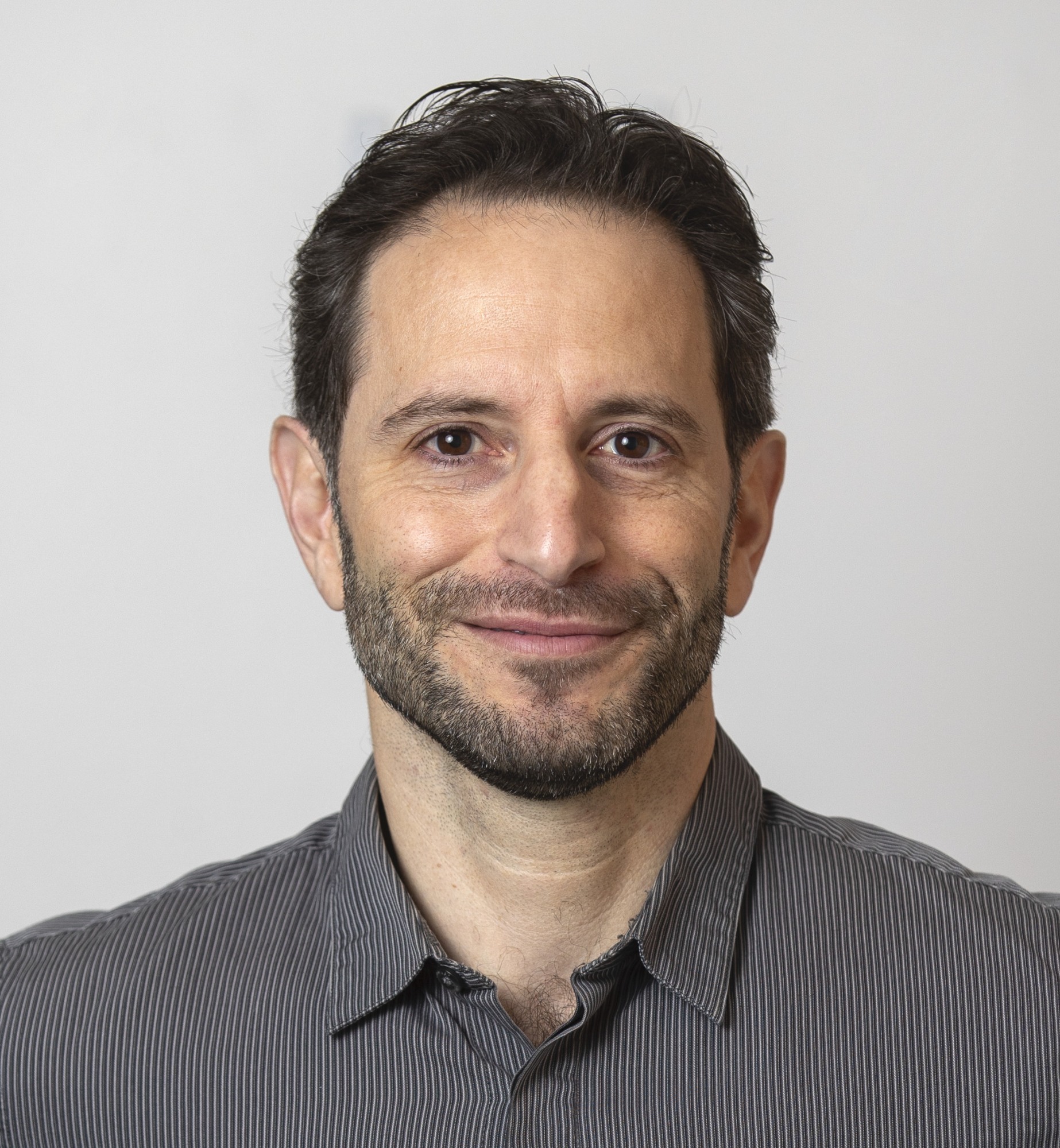
“Our results provide a fresh perspective on the source of working memory deficits in dopamine-related disorders,” said Frank.
Frank has spent years creating computer models that map the complex brain architecture and mechanisms that make working memory possible. His models depict the prefrontal cortex and basal ganglia, the regions of the brain responsible for memory. Frank and his lab have shown that working memory relies on a mechanism called input and output gating to first store, then retrieve, information. His lab has also shown how mechanisms that run the brain’s dopamine delivery system help reinforce this process: emphasizing which pieces of information are important to store and therefore contributing to the brain’s ability to learn.
In developing the lab’s newest model, Soni was inspired by a 2018 human experiment Frank conducted in concert with Carney affiliate Matt Nassar, an assistant professor of neuroscience. In that study, Frank and Nassar established that participants learn to “chunk” related pieces of information together when storing them in order to conserve space in their working memory.
Interested in whether she could model this chunking process, Soni incorporated an information compression mechanism into Frank’s computer model and then challenged it with the working memory task used in the previous human chunking experiment. The model replicated the human results: over the course of the experiments, it learned to adopt a chunking strategy.
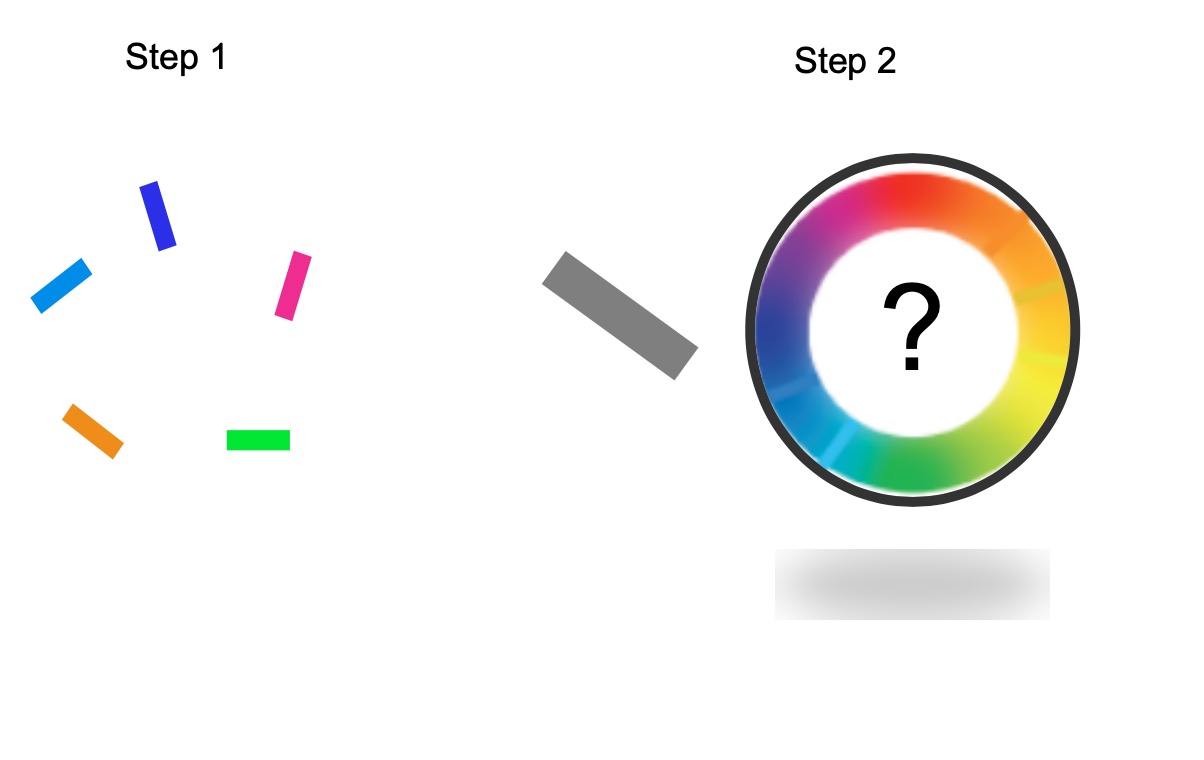
To prove that the model could learn to chunk the way people do, Soni showed the model a screen with different colored blocks oriented in different directions. Afterwards, the model was asked to recall which colored block was pointing in which direction.
The results showed that as the number of colored blocks increased, the computer model began “chunking” together similar colors, such as blue and light blue.
One of the reasons computational modeling is so valuable for neuroscientists is that, unlike in a human experiment, researchers can observe exactly how the mechanisms they have built behave in experimental settings. Soni could see what the new model was doing when it replicated the human experimental results. Over the course of a number of trials, the model learned that it should chunk related colors together to conserve space. This learning process involved changing how it was input gating blue and light blue. When it was doing a better job of recalling the orientation of a larger number of blocks because it had chunked those similar colors together to save space, the model’s dopamine mechanism kicked in, telling the model to continue to use this strategy when faced with the same set of constraints in subsequent trials.
The lab’s simulations with the new model, for the first time, point to learning – rather than capacity – as the real limitation and driver of working memory. Soni established this by also running the trials on a model without the ability to chunk, but with plenty of space to store items. She found that while the model with the chunking mechanism was able to strategically store information to its full storage capacity, the model without the chunking mechanism did not even realize it had access to such a large amount of storage and was worse at both storing and retrieving the items.
In another part of the experiments, Soni altered the model’s dopamine delivery system to emulate, one at a time, what is known about dopamine levels in patient populations including Parkinson’s disease, schizophrenia and ADHD. When she challenged the model to the same series of trials, the results showed that, without a healthy dopamine delivery system, the model was less able to learn and strategize across trials to the same extent. The model was not learning how to use its storage space as efficiently and was not chunking items as much.
Frank said this increased understanding of what takes place within the basal ganglia and thalamus for these patient populations may spur clinicians to adopt different treatment options.
“Take Parkinson’s disease as an example,” he said. “Most people think of it as a movement disorder because changes in movement are so obvious. But it turns out that Parkinson’s patients also have changes in working memory. People with the disease are generally treated with drugs that target the prefrontal cortex, but our new findings suggest that we should be testing whether drugs that target the basal ganglia and thalamus help to improve symptoms.”
This research was supported by ONR MURI Award N00014-23-1-2792 and NIMH R01 MH084840-08A1. Aneri Soni was supported by NIMH training grant T32MH115895. Computing hardware was supported by NIH Office of the Director grant S10OD025181.